Lots of interesting abstracts and cases were submitted for TCTAP 2024. Below are the accepted ones after a thorough review by our official reviewers. Don¡¯t miss the opportunity to expand your knowledge and interact with authors as well as virtual participants by sharing your opinion in the comment section!
TCTAP A-037
Coronary Angiography Deep Learning Model for Diagnosis of Neoatheroslcerosis
By Yingqian Zhang, Changfu Liu, Yun-Dai Chen
Presenter
Yingqian Zhang
Authors
Yingqian Zhang1, Changfu Liu1, Yun-Dai Chen1
Affiliation
Chinese People's Liberation Army General Hospital, China1
View Study Report
TCTAP A-037
Left main
Coronary Angiography Deep Learning Model for Diagnosis of Neoatheroslcerosis
Yingqian Zhang1, Changfu Liu1, Yun-Dai Chen1
Chinese People's Liberation Army General Hospital, China1
Background
Routine diagnosis of NArequires OCT during CAG follow-up. However, the use of additional contrast in OCT leadsto high risk for patients with contrast-induced acute kidney injuryor contrast allergy. Moreover, OCT is not appropriate forassessment of heavily calcified, tortuous, ostial left-main lesions, and angulatedanatomies. In addition, the high cost of OCT is a potential limitation bypracticing interventional cardiologists In addition, the high costof OCT is a potential limitation by practicing interventional cardiologists. Those limited the expected effects of OCT-guided NAidentification and treatment. Compared with OCT, CAG is a cost-effective andwidely available imaging. Therefore, an accurate deep learning method based onCAG may have the potential to assist NA diagnosis.
Methods
This was a single-center, diagnosticstudy that used CAG images from Chinese PLA General Hospital in China.Diagnosis of NA using OCT was considered as the gold standard. InstitutionalReview Board (IRB)/Ethics Committee approvals were obtained in Chinese PLAGeneral Hospital. All eligible participants provided their written informedconsent before intracoronary imaging. This study complies with the Declarationof Helsinki and was registered at http://www.clinicaltrials.gov (NCT04220437). We included 123 consecutive in-stent restenosis patients with optical coherencetomography during angiography follow-up after percutaneous coronaryintervention. A total of 126 lesions from the enrolled patients were randomlyselected as the training cohort (85 lesions) and testing cohort (41 lesions).We adopted Inception V3 as the main network, and added two convolutionallayers, a pooling layer and a fully connected layer. To further promote thediagnosis performance, we fused the deep learning signature with relatedclinical features to construct DeepCAG. For comparing theperformance of DeepCAG with practicing interventional cardiologists, anindependent CAG dataset consisted of a total of 41 lesions from 41 ISR patientsincluding 15 NA and 26 NNA patients were employed. We enrolled five practicing interventionalcardiologists to participate the comparison study. Three metrices: the accuracy of the overall lesiondiagnosis, the accuracy of NA identification (sensitivity), and the accuracy ofNNA identification (specificity) were used to evaluate the performance ofdifferent group cardiologists in diagnosing NA.
Results
Of allenrolled 126 lesions,78 were categorized as NNA (61.9% of total lesions), and 48were categorized as NA (38.1% of total lesions), respectively. Baselinecharacteristics among the 2groups are listed in Table 1. The mean time intervalfrom stent implantation to OCT examination was longer in NA group than the NNA And the deep learning signature was able to distinguish NA with 0.867sensitivity, 0.462 specificity, and AUC of 0.697 on the testing set. Furthermore,the class activation map was used to visualize the region of interest of thedeep learning model on the CAG image. After deep learning signature are fused withclinical features, the performance of the DeepCAGmodel is further improved, and the AUC is improved to 0.751. The predictionaccuracy of NA and NNA patients is more balanced (sensitivity: 0.733,specificity: 0.692) compared with deep learning signature (sensitivity: 0.867,specificity: 0.462).An independent test dataset of 41 lesions (15NA, 26 NNA) CAG images was used to compare the performance of DeepCAG with interventional cardiologists in differentiatinglesions with NA (Table3). The accuracy of DeepCAG is 0.707. The accuracy ofsenior cardiologists is 0.659 and 0.634, which is comparable to that of DeepCAG(p =0.416, p =0.316, respectively). The accuracy of the juniorcardiologists is 0.561, 0.610, and 0.463. The accuracy of DeepCAG is superiorto the junior cardiologists, but the difference is of no statisticalsignificance (p =0.181, p =0.337, p =0.056, respectively).The sensitivity of DeepCAG is 0.733, which is comparable with both seniorcardiologists and junior cardiologists.The specificity of the DeepCAG is 0.692, which is comparable with the senior cardiologists(0.692, 0.692, p =1.00, p =1.00). However, it is relativelyhigher than the junior cardiologists.
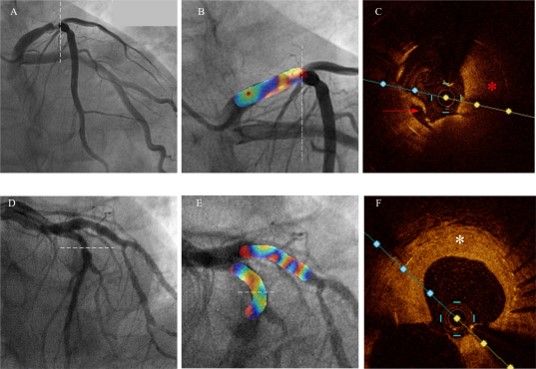
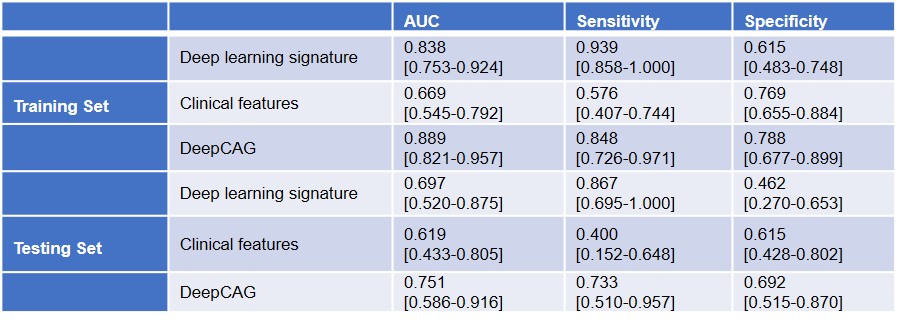
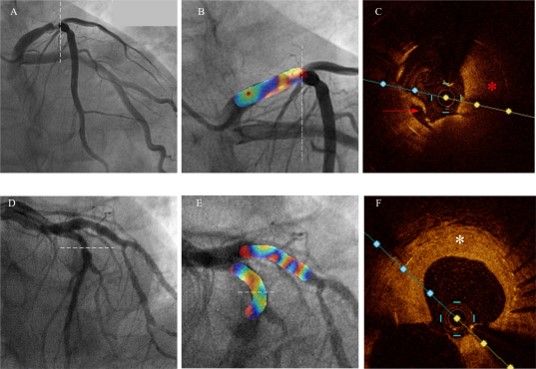
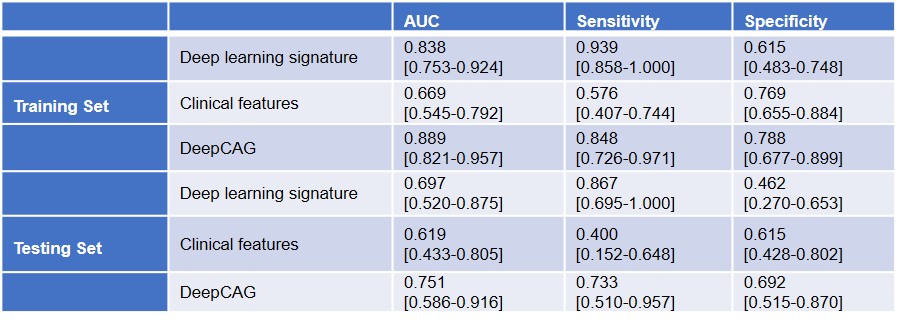
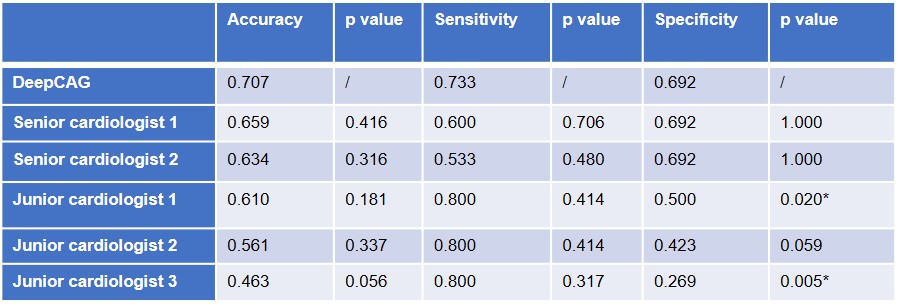
Conclusion
Thisstudy focused on developing a deeplearning-based nomogram model (DeepCAG) based on CAG images and clinical featuresfor NA diagnosis. It could diagnose NA from other mechanismsin ISR patients independent of OCT. The diagnostic accuracy of DeepCAG on NA is comparable to that of the senior cardiologists,but it significantly improved the specificity in junior cardiologists. The DeepCAG is designed to assist cardiologists as an efficientscreening tool so they reduce the diagnose workflow time, limit the use ofcontrast, and allow them to NA diagnosis in lesions where OCT images cannot beacquired. The precise NA diagnosis has the potential to guide the treatmentstrategy for patients. We constructed DeepCAG to assist NA diagnosis throughcoronary angiography. Some regions that the deep learningsignature pays attention to are different from the imaging signs commonly usedby cardiologists, but they show the ability toidentify NA comparable to senior cardiologists , which means that thedeep learning signature can explore the information in the CAG images that aredifficult capturing for the naked eye. Although the sensitivity of DeepCAG iscomparable with both senior and junior cardiologists, it improved the specificityof NA diagnosis in junior cardiologists. Science NA may not favor from DCBdilation and ELCA during reintervention, it has the potential to reduce thenumber of unnecessary procedures.Based on our study, we make tworecommendations for the implementation of DeepCAG into clinical practice.First, our model shows advantages in improving the specificity of NA diagnosis,especially for junior cardiologists. The OCT image acquisition andinterpretation is subject to interobserver variabilities. DeepCAG could providea consistent second opinion on NA diagnosis, particularly for juniorcardiologists. Second, in non-academic centers, lesions with ostial or tortuousanatomy, patients with severe renal or cardiac dysfunctions, OCT images cannotbe acquired. DeepCAG can give a quite accurate NA diagnosis and assist tailoredre-intervention strategy and medication therapy.